In the last edition of Money on the Table, I talked about the huge potential for hybrid pricing models in SaaS. In this edition, I will showcase how this would work in practice.
Here’s the TL;DR of the previous edition: by combining pricing models you can (1) increase your average contract value, (2) shorten the deal cycle, and (3) improve the predictability of your revenue. Resulting in both faster growth and higher valuations.
Since everyone is familiar with OpenAI, and perhaps even its struggles with pricing, we’ll examine what a pricing redesign could look like for them.

Kyle Wiggers in TechCrunch

First, we’ll discuss the strengths and shortcomings of the existing pricing model at OpenAI. Next, we’ll identify the requirements for a new model. And finally, I’ll suggest a first draft OpenAI could validate.
Disclaimer: B2B pricing is 50% art and 50% science. Since I don’t have access to OpenAI’s data, this is purely an intellectual exercise. Ideally, you want the data to point you in the right direction and then validate with new customers before rolling it out.
Existing Model: Strengths and Shortcomings
When designing your pricing strategy from scratch, all you have are assumptions and gut-feel. But once you have paying customers, you start to validate or invalidate your assumptions. Earlier this year, Sam Altman openly admitted that the current pricing model is broken. Primarily because the computing power is more costly than the $200 monthly fee they charge for Pro subscriptions.
As of today, ChatGPT offers the following pricing plans:
ChatGPT Plus: $20/month for individual users.
ChatGPT Pro: $200/month for professionals requiring advanced features.
ChatGPT Team: $30/month per user (or $25/month with annual billing) for collaborative environments.
ChatGPT Enterprise: Custom pricing tailored for large organisations.
Based on the information I have access to, here are the strengths and shortcomings of OpenAI’s existing pricing model.
Strength #1
Since they opted for a seat-based model, their revenue is highly predictable. Future revenue is much less predictable with usage-based or out-come based pricing. Predictability is generally rewarded by investors and buyers with a higher multiple.
Strength #2
In Q4 ’24, CFO Sarah Friar reported that roughly 75% of revenue comes from consumers. These customers convert directly on the pricing page using a credit card. The other 25% comes from business users, educational institutions, and API usage. Even a substantial part of this group will convert without human interaction.
Shortcoming #1
As Altman admitted, OpenAI is losing money on a large number of customers. This is simply because there is no variable tied directly to the usage. They might have a fair-usage clause in the contract or in-product caps, but this only limits usage. You never want to limit usage.
Shortcoming #2
The Business plan is too simplistic. When a buyer is looking for 2 or 3 seats, they’ll happily pay the $30/month. But when a prospect is looking to purchase 50 or 100 seats, they’ll demand a discount. Because there is only 1 pricing metric, any discount you provide is given on the total amount. When you have multiple pricing metrics (e.g. platform fee, usage fee, cost-based), you can negotiate 1 line item that makes up a part of the quote.
Shortcoming #3
The value creation and value capturing are not 100% aligned. ChatGPT’s value creation is two-tiered. First, it'll provide answers to your queries. Second, for every query you submit –plus the context you provide– the quality and relevance of the answers improve over time.
Right now, it’s mainly monetising the first part.
To summarise, OpenAI is losing money mainly because its pricing model is too simplistic. The new pricing should cover cost more adequately. At the same time, since the majority of purchases happen without human intervention, it needs to be simple.
“OpenAI is losing money because its pricing model is too simplistic”
From Simplistic to Simple Pricing
Based on the strengths and shortcomings of OpenAI’s current model, these are my specs for a new pricing:
Must cover all usage expenses
Must be simple
Value-based
Increase ARPU / ACV
The new pricing should have a metric directly tied to usage to stop the company from bleeding money. It has to be simple, otherwise online conversion is likely to drop. Additionally, to avoid a race down the bottom and perhaps even create a lock-in effect, OpenAI would benefit from emphasising the quality improvement and personalisation over time. In terms of pricing, this means monetising usage separately from access to a personal environment. And finally, what’s the point of a new pricing strategy if you can’t increase your revenues.

Sam Altman discussing credit-system
Altman’s post on X earlier this month, shows he’s already thinking along similar lines. However, his remark suggests a complete shift to usage-based with a minimum monthly fee. Instead, introducing an additional layer to the pricing and communicating it more strategically would improve its success chance. After all, OpenAI is famous for having the most confusing API pricing in the world. We don’t want another such a debacle.
Here’s a rough outline what I would do.
Plus and Pro:
Lower the seat-based fees substantially. The upside: seemingly more competitive pricing and lower barrier to get started.
Position the subscription as your private environment that learns from your queries and improves over time.
Introduce a charge per query type. Ideally not more than 3 different prices.
Leverage user data to determine price points. It’s generally a good practice to let power users heavily subsidise light users. Assume you want to earn >50% from the top 20% users.
Optional: since consumers aren’t as sophisticated buyers as B2B, you might want to work with top-up credits.
Team and Enterprise:
Lower the seat-based fees substantially. The upside: seemingly more competitive pricing and lower barrier to get started.
Add a platform fee for XK/year and XXK/year. This is for your company environment that gets smarter over time, but also the price for excluding user data from the LLM. The upside: stimulates user adoption and decreases cost per user for buyer.
Add a charge per query type. Again, max it at 3 different types (group some if necessary). Use a credit system to stimulate more usage.
Don’t ever connect credits to data memory, processing time, or any other technical variable. The value is in answering a query. No one cares about your cost.
Provide the option to install soft caps: notifying billing contact that certain monthly threshold is reached.
As always, how you charge is a lot more important than how much you charge. You can leverage user data to estimate price points and tweak price points while you learn. What you cannot do is change the packaging every month.
If executed correctly, you can increase the ACV by >20% fairly easy this way without being perceived as unfair. Power users will end up paying more than light users and it’ll be easier to get started.
Want to learn more about SaaS pricing and packaging? Follow Money on the Table or schedule a free consult at https://revfixr.com/contact
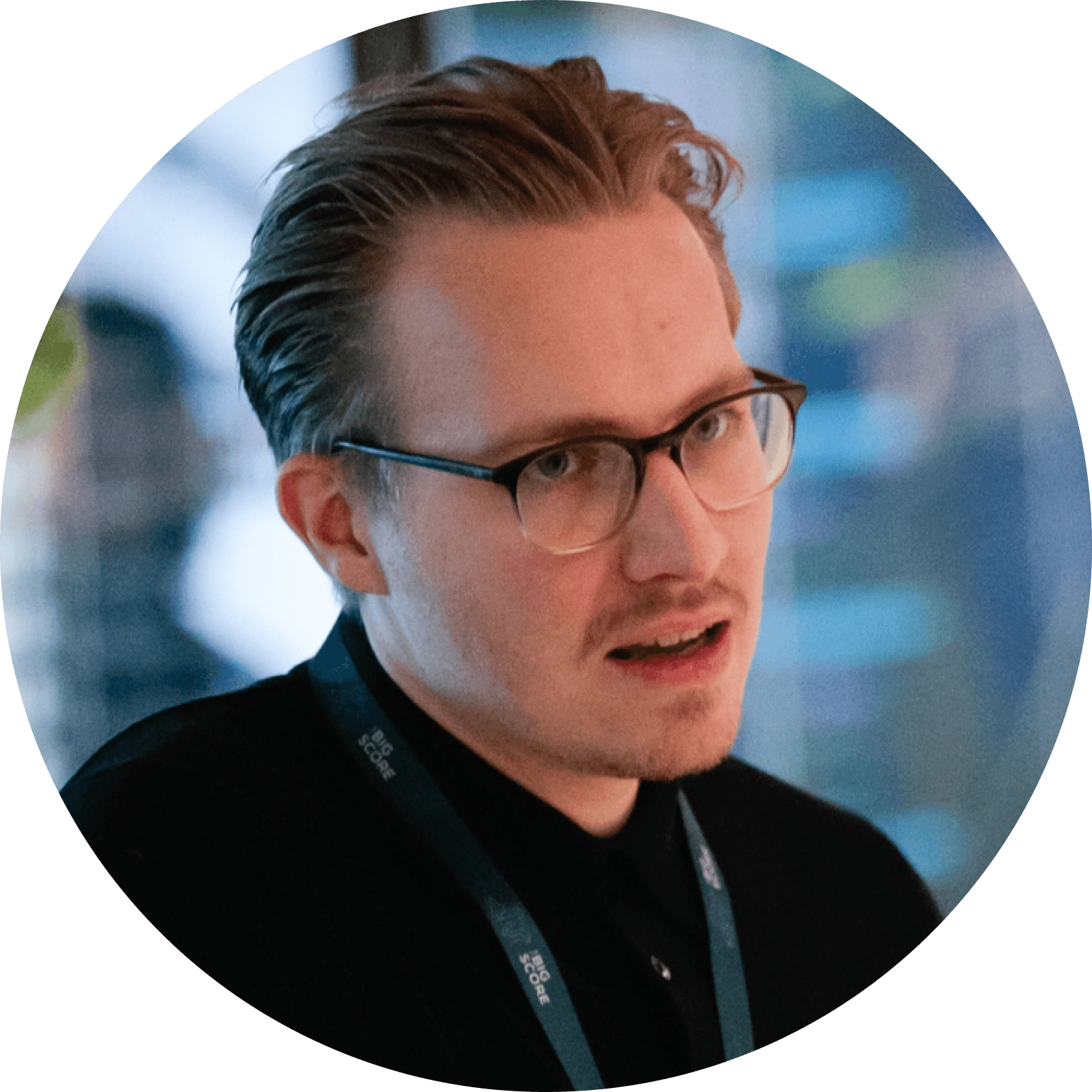
Tjitte Joosten is the Founder of RevFixr, the one-stop shop for better monetisation of your customer base. RevFixr turns pricing into your biggest growth lever. Prior to founding RevFixr, Tjitte was responsible for the commercial strategy and operations at tech companies like Docfield and Experfy.